Since the announcement of the 4th Industrial Revolution in 2011, multitudes of emerging technologies have transpired around “Industry 4.0”. Technologies such as digital twins, Industrial Internet of Things (IIoT), and cyber-physical systems have come into the scene as core elements, providing the necessary ingredients for a paradigm shift in manufacturing. Technologies around predictive analytics and artificial intelligence are pioneering new approaches with many use cases, including predictive maintenance, autonomous process optimization, and pattern recognition – used to identify potential failures in real-time. Because of this, data science is becoming an invaluable discipline for our industry, serving to transform information into knowledge. As data scientists begin to play a fundamental role in the new way of understanding industrial and manufacturing activities, a new challenge appears: data engineers have to deal with unknown processes, procedures, operations, science and specific casuistries (where all of the potential answers lie).
Every industry requires different machine learning and deep learning systems. The retail sector is very customer oriented, and opportunities lie behind the buyer's preferences and needs. Natural language processing and media analytics are tools widely used to make attractive suggestions to the final consumer. Fintech requirements are also different. Data scientists must adapt to specific algorithms for fraud detection, aggregation, and anomaly detection of tones of commercial transactions in real-time or for insurance policies based on a large variety of medical and health risk indicators.
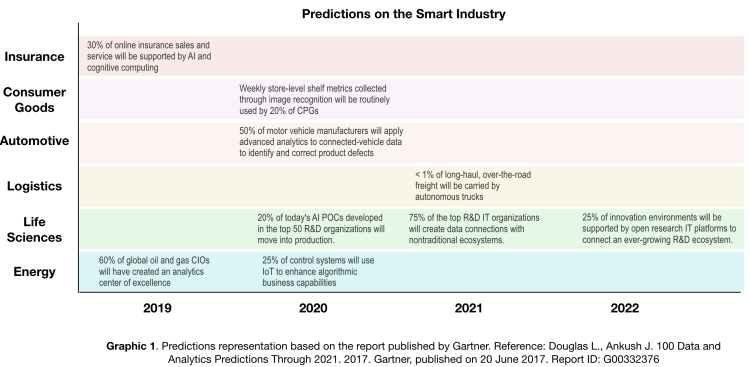
The manufacturing activity for Industry 4.0 is even more complex. The pharmaceutical and biotech industries are a special case among the wide manufacturing spectrum. Medicine prerequisites like safety, quality, and efficacy must be validated before a product goes to market. The linkage between health agencies and business requirements is a unique intersection that makes the pharma ecosystem special, the reason being that human safeguards must always be the top priority. When processes are designed and implemented in the factory, they are subject to strong quality controls based, in many cases, on the assumption that variability must be minimized. This makes it difficult to validate operations that supply results based on algorithms like the random forest. However, some machine learning algorithms have been accepted by the European Pharmacopoeia as valid chemometric techniques for processing analytical data sets.
As we move forward, the Administration and the Regulatory Institutions are cautiously observing and establishing the first approach to the set of laws that must govern the data, processes, and the results obtained through AI algorithms. Thus, a new generation of data scientists and technological experts is emerging, with a clear orientation towards regulatory guidance, initiating the path to a commonly accepted approach to data management and data integrity. When results coming from AI algorithms are used in aeronautics, automotive, medical, and pharmaceutical activities with direct impact on people’s health, the full process must be surveilled and understood in order to grant personal safety.
The unique characteristics in Industry 4.0 require that manufacturing specialists take care not only with the products being manufactured but also with the associated data and analytics offered as an added value to the distributed goods. 4.0 industrial data scientists must know the algorithms to apply on top of the data ecosystem, which requires future innovators to be multidisciplinary and specialized at the same time. They have the important mission of satisfying the growing demand for data science services applied everywhere.